genome-wide scans for variants under selection
applying machine learning and computer vision methods to images of genetic data
This was a project as part of my dissertation thesis
Building on our work leveraging ancestry patterns to detect and characterize signatures of rapid adaptation in admixed populations, I am currently working on developing methods to improve our ability to study the evolutionary history of admixed populations.
I applied machine learning and computer vision methods to images of admixed chromosomes painted by genetic ancestry. Typical population genetics methods rely on one or more summary statistics. This image-based appraoch allows us to leverage all the information provided by genetic ancestry.
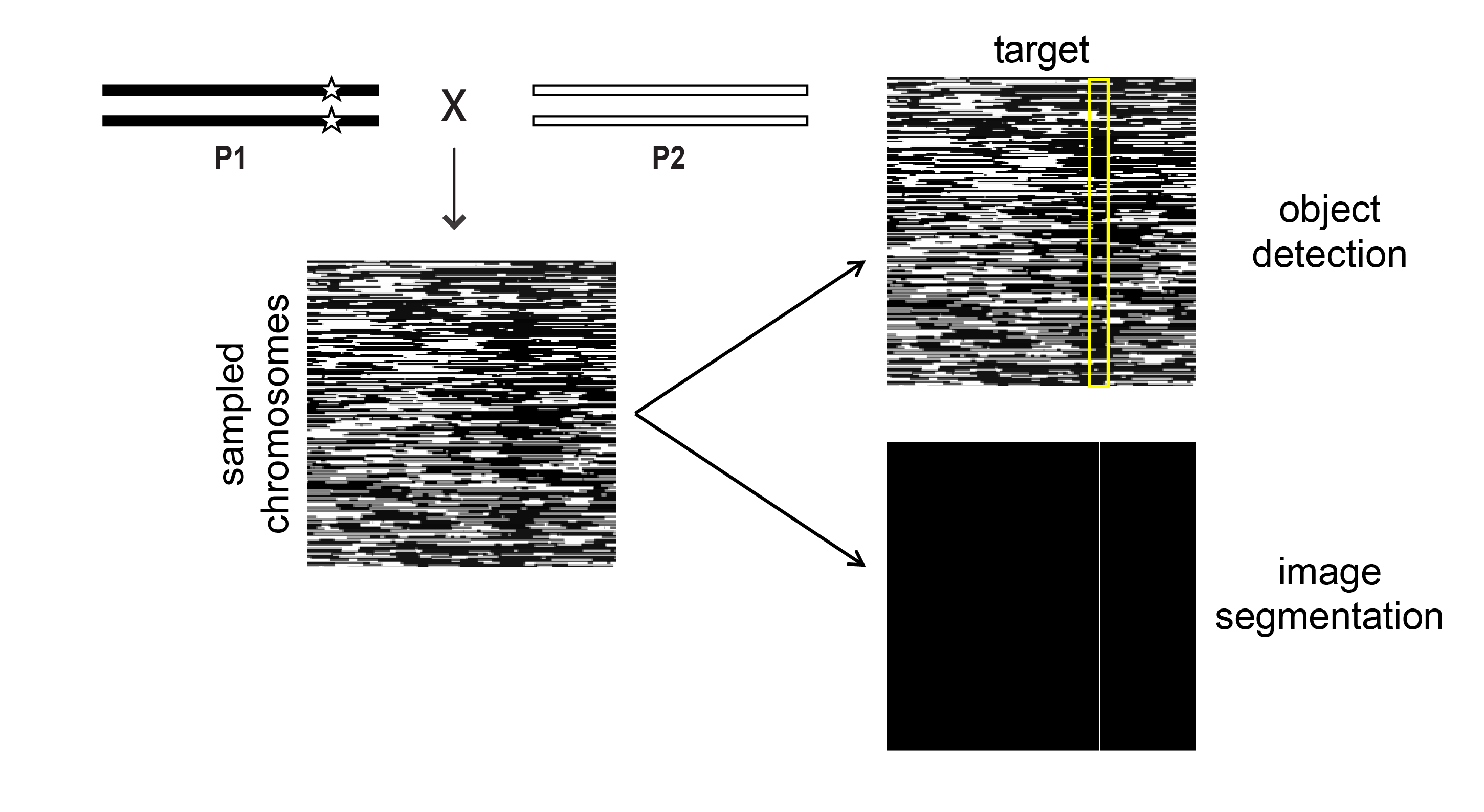
In addition to optimizing these methods for this type of data, I also identified the timescales, genomic features, and demographic/selective histories that are best suited for this ancestry-based detection of genetic variants of interest through simulations. This work was published in 2023 in MBE.